Resistance Is Futile, But Maybe Not With AI
Your job is on the line, especially if artificial intelligence gets smart enough to answer these 6 questions.
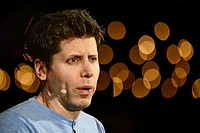
(Bloomberg Opinion) -- The novelist Neal Stephenson’s prophetic power never ceases to astonish. e (1995), is set in a technologically highly advanced world, with ubiquitous nanotechnology in addition to something strangely familiar called “P.I.” The abbreviation is explained in the following exchange:
“I'm an engineer … Did some work on this project, as it happens.”
“What sort of work?”
“Oh, P.I. stuff mostly,” Hackworth said. Supposedly Finkle-McGraw still kept up with things and would recognize the abbreviation for pseudo-intelligence, and perhaps even appreciate that Hackworth had made this assumption.
Finkle-McGraw brightened a bit. “You know, when I was a lad they called it A.I. Artificial intelligence.”
Hackworth allowed himself a tight, narrow, and brief smile. “Well, there's something to be said for cheekiness, I suppose.”
Yet, for all their technological sophistication, these two men are “neo-Victorians.” They have chosen to embrace 19th-century manners and fashions partly as a defense against the collapse of state power and the fragmentation of nations into “phyles,” i.e., tribes.
There is an important warning here for everyone giddy with the recent advances of generative AI. Breathtaking developments in the realm of technology do not render history obsolete. It lives on alongside the latest gadgetry, because the present is not where history ends and the future begins; it is where the past and the future fuse.
Since OpenAI released ChatGPT on Nov. 30, 2022, the world has been in an AI frenzy. Within just five days, ChatGPT had over one million users. Now there are 100 million. The company’s latest triumph is Sora, which can churn out DreamWorks-quality animation in response to your most whimsical prompt. All of this is made possible by deep-learning systems with hundreds of billions of parameters, a term defined here. (To give an example, GPT-4’s training model needs more than a trillion parameters.) And the amount of computation used to train state-of-the-art large language models (LLMs) is more than doubling every six months.
Over time, this speed of increase in the size of the models must slow and converge with Moore’s Law (see below), or the price of training new models will become exorbitant — out of any plausible relationship to the money that might be made from commercializing the technology. That means AI can advance only so long as computing power keeps getting exponentially cheaper.
I have six questions about the technological, financial and economic future of AI:
In 1965, Gordon Moore, the co-founder of Intel Corp., speculated that that the number of components (transistors, resistors, diodes or capacitors) in a dense integrated circuit — otherwise known as a microprocessor, semiconductor or chip — would double every year for the next 10 years. On the basis of further observation, he revised that to every two years in 1975. Moore’s Law has held up to nearly 50 years of innovation.
It now seems clear that Moore’s Law is a manifestation of a deeper phenomenon that long predated Moore, who died in 2023, and is likely to outlive him for many years. Price-performance for computation (the number of operations a computer or chip can perform per dollar of cost) and for other related processes such as information storage and information transportation has been increasing exponentially since the late 19th century, when Herman Hollerith’s proto-computers used electromechanical relays and punch cards. Even if there are notional limits to what the current silicon-based technology can achieve, we should assume that a new technology will soon be found when those limits are reached. Let’s not bet against Moore’s Law.
In the first instance, it must be Nvidia Corp. — and Taiwan Semiconductor Manufacturing Co. They hold the dominant positions in the design and manufacture of the crucial graphics processor units (GPUs) on which LLMs run.
Who else makes money? OpenAI for sure, because its GPT-4 is a (if not the) state-of-the-art LLM, and it has the deep pockets of Microsoft Corp. to finance the training of the next version, presumably GPT-5. Today, the world hangs on every word uttered by OpenAI’s chief executive, Sam Altman. But OpenAI faces more immediate competition than Nvidia, and not only from Alphabet Inc.’s Google. Open-source AI systems including LLaMA, Alpaca and Vicuna are not so far behind. It is easy to imagine a significant proportion of users being willing to settle for AI chatbots that are not quite so great but free (or ad-supported), leaving the professionals to pay for the premium service.
Financial history is full of examples of disruptive technologies begetting equity market bubbles. Could the current AI mania be another?
There are certainly many bogus companies that have recently added “AI” to their pitch docs, just as there were many in the 1990s that spuriously claimed to “dot-coms.” But is Nvidia a bubble stock? As my Bloomberg Opinion colleague John Authers recently pointed out, Nvidia is performing more impressively in terms of sales and margins than its equivalent in the dot.com era, Cisco Systems Inc., which made the routers on which the internet ran.
Huang calls AI “probably the single greatest invention of the technology industry” and “likely … the most important thing of the 21st century.” But we’ve heard such hype before. The reality is (ask Tesla Inc.) that wherever there is the prospect of outsized profits there will very soon be competition. Google, Microsoft, Amazon.com and Advanced Micro Devices Inc. are all designing their own AI chips. And, under the leadership of Pat Gelsinger, Intel is reemerging from two decades of missed opportunities, aiming to compete in both design and the foundry business.
The way bubbles burst is partly that competition inexorably drives down margins, making a nonsense of peak valuations. But there is an additional dynamic. On one side, the amounts being invested in AI are colossal. Not content with the $13 billion OpenAI received from Microsoft, Altman now talks of needing $7 trillion for chip manufacture and power generation. Impressive though GPT-4 and Sora are, there must come a point when even OpenAI’s prospective revenues cannot justify such immense capital sums. Fact: $7 trillion is the total annual budget of the federal government proposed by the Biden administration for the 2025 fiscal year.
Earlier this month, The Information published some significant intimations of doubt in corporate America about just how much LLMs can really do for their businesses. During a February earnings call, Amazon CEO Andy Jassy said that near-term revenue from AI was “relatively small.” Salesforce Inc. executives said that generative AI wouldn’t make a “material contribution” to revenue growth this year. These are the first signs of a hype cycle passing its peak.
A key concern is that AI still “hallucinates” (i.e., makes stuff up) much more than humans do. The real determinant of market adoption is what AI can do with human-or-better reliability.
AGI refers to a hypothetical generalized algorithmic system that can match generally educated humans at all cognitive tasks.
This is clearly what Altman aspires to build. But is it attainable? That is really a question about how fast we can grow computational capacity to the scale likely needed to compete with the human brain — which might mean a 100-trillion parameter training model, compared with GPT-4’s estimated one trillion. That would require a whole lot of computing power (or “compute,” as AI mavens like to say).
In 1999, the futurist Ray Kurzweil predicted that AGI would come by 2029. More recently, on Jan. 1, 2022, the weighted average of forecasters compiled by the aggregation platform Metaculus projected that “weak AGI” would reach human levels of general intelligence in 2042. Three months later, Google’s PaLM AI mastered “chain-of-thought reasoning,” which was seen as a key obstacle.
The most recent Metaculus average of 1,170 forecasters is that “the first weakly general AI system will be devised, tested and publicly announced” in 2026, with an interquartile range between 2025 and 2030. It will take an additional 28 months beyond the release of “weak AGI” for AGI to become superintelligent, i.e., smarter than even the best humans at all cognitive tasks.
Such is the power of exponential growth. If model sizes keep doubling every six months, quadrupling every year, and increasing eightfold every 18 months, then even a 10-fold underestimate of the amount of computational power required for AGI would delay the expected arrival time by just 19 months. A 100-fold underestimate would delay it by just three years.
AGI is the stuff of science fiction (think of HAL 9000 in Arthur C. Clarke's ). That does not mean it cannot become fact, like the nanotechnology and talking books of .
For three centuries, the labor market has adapted to new technology, and the long-term trend has been toward increasing aggregate employment, hourly productivity and, ultimately, wages. New technology does destroy certain occupations: handloom weaver, stenographer, telephone operator, elevator operator. But those jobs all went away without protracted mass unemployment. With new technology come other occupations.
McKinsey & Co. research estimates that, even without generative AI, automation could take over tasks that account for 21.5% of the hours currently worked in the US economy by 2030. With AI, the share is 29.5%. It would be nice to imagine everyone merrily enhancing their productivity with the help of an AI co-pilot. It seems more likely that white-collar employment will shrink right across the board from the legal profession to entertainment.
With the advent of AGI, even more radical outcomes are imaginable, in which it could cease to make sense to employ humans in most roles, including even enterprise leadership.
You know the answer to this one: Yes.
Technological change has a long history of causing political controversy and social disruption, but organized resistance has rarely succeeded in preventing adoption. Violent riots in London in 1675 and Leicester in 1773 saw textile workers destroy the machines that they feared would destroy their livelihoods. Starting in 1811, gangs of Luddites sabotaged and smashed power looms.
There are Luddites in our time, too. In December 2022, Apple Inc. introduced a service allowing book publishers to use human-sounding AI narrators, threatening to displace the voice actors who record audiobooks. The prospect that generative AI might one day replace screenwriters and actors became the central dispute in the Hollywood WGA and SAG-AFTRA strikes last year.
Resistance to technological change is generally pretty futile. The Luddites, though quite well organized, were crushed. Today, only 1.3% of US workers in financial services and 2.3% of workers in professional and business services are unionized, below the national average of 10%. With this lack of organization, the losers of the AI revolution will stand little chance of resisting in the workplace.
However, political resistance to a new technology can succeed if the public and the elites take fright. A good example was the backlash against human cloning and genetic engineering. In 1997, the creation of “Dolly the sheep” in the UK marked the first successful cloning of a mammal using somatic cell nuclear transfer. The US passed the Human Cloning Prohibition Act in 2003, prohibiting both reproductive cloning and research cloning using federal funds.
This history raises the possibility that, as generative AI becomes more human-like, regulators and legislators may come under pressure to restrict its use — for example, in creating deepfake video or audio content impersonating an individual.
In Neal Stephenson’s imagined Diamond Age, PI has failed to achieve that level of mimicry, but only because, “after all of our technology, the pseudo-intelligence algorithms, the vast exception matrices, the portent and content monitors, and everything else, we still can’t come close to generating a human voice that sounds as good as what a real, live ractor [role-playing actor] can give us.”
In our Silicon Age, AI has easily overcome that obstacle. But can regulation — or a more visceral popular backlash — slow the exponential growth of compute, the rise of the LLMs, and the fateful approach of AGI? In a future column, I’ll turn to the emerging politics of AI.
More From Niall Ferguson at Bloomberg Opinion:
- Global Population Crash Isn't Sci-Fi Anymore
- If You Think World War III Is Unimaginable, Read This
- The US and Europe Risk Flunking Geopolitics 101
This column does not necessarily reflect the opinion of the editorial board or Bloomberg LP and its owners.
Niall Ferguson is a Bloomberg Opinion columnist. He is the Milbank Family Senior Fellow at the Hoover Institution at Stanford University and the author, most recently, of “Doom: The Politics of Catastrophe.”
More stories like this are available on bloomberg.com/opinion
©2024 Bloomberg L.P.